Plenary talks
|
|
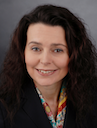
Maria Kateri RWTH Aachen University Germany
|
Title: Step-Stress Accelerated Life Testing Models: Statistical Inference and Optimal Experimental Design
Abstract: Accelerated life testing (ALT) is widely used in reliability analysis across a range of fields, from material sciences and quality control to biomedical sciences and ecology statistics. Step-stress models form an essential part of ALT. In a step-stress ALT (SSALT) model, test units are subjected to gradually increasing stress levels at intermediate time points throughout the experiment. Statistical inference is then developed to estimate parameters such as the mean lifetime under each tested stress level. Parameter estimates under normal operating conditions can be derived by using a link function that connects the stress levels to the corresponding parameter of the assumed lifetime distribution. Respective statistical models are specified according to the assumptions made regarding the time points of stress level change, the experiment’s termination point, the underlying lifetime distributions, the type of censoring (usually present), and the way of monitoring failures.
We explore SSALT models and their adaptability in various experimental setups. Our focus is on a model that employs a general scale family of distributions, offering flexibility and leading to explicit expressions for the maximum likelihood estimators of the scale parameters in the underlying lifetime distributions. The approach is demonstrated for Type-I censored experiments, considering both continuous and interval monitoring of the test units. We address maximum likelihood, maximum product of spacings, as well as Bayesian estimation. Additionally, we discuss optimal experimental design for SSALT experiments. Finally, we consider SSALT modeling in heterogeneous populations, where test items are grouped according to their aging behavior. In such cases, heterogeneity is captured using a mixture model approach.
|
|
|
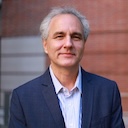
Enrique Lopez Droguett UCLA USA
|
Title: Quantum Computing for Risk and Reliability: Outlook & Opportunities
Abstract: Industry 4.0, combined with the Internet of Things (IoT), has ushered in the requirements of risk, reliability, and maintainability systems to predict physical asset’s performance and aid in integrity management. State of the art monitoring systems now generate large amounts of multidimensional data. Moreover, customers are no longer requiring that their new asset investment be highly reliable; instead, they are requiring that their assets possess the capability to perform fault diagnostics and prognostics and provide alerts when components need to be intervened. With this new Big Data at the engineer’s fingertips, more sophisticated methodologies to handle this data have been developed and expanded within the risk and reliability (R&R) field. Indeed, in the past decade, the availability of powerful computers and special-purpose information processors have led to the development and application of machine and deep learning models for the assessment of R&R of complex engineering systems (CES) that can identify multifaceted and subtle degradation patterns in monitoring data.
In recent years, a new computing paradigm has gained momentum: quantum computing, which encompasses the use of quantum mechanical phenomena to perform computations. The power and flexibility of a quantum computer comes from the use of qubits that have the ability to be in a superposition state, or multiple states at once, and share entanglement with each other. By leveraging on these properties, quantum computers can perform operations that are difficult to achieve at scale in classical digital computers. This opens the door to new exciting opportunities for the design and performance assessment of complex engineering systems in general, and for the development of new quantum methods for R&R that might be able to recognize intricate interdependent scenarios and components as well as multilayered degradation patterns in CES from multidimensional monitoring data that classical machine learning approaches cannot.
In this lecture, we discuss the main concepts underpinning quantum computing and its advantages, disadvantages, and potential impact on the risk and reliability assessment of CES. We present state-of-the-art quantum optimization, quantum inference, and machine learning algorithms for developing predictive solutions for risk and reliability assessment of complex engineering systems. We then examine potential opportunities, limitations, and challenges for the future development and deployment of quantum computing based risk and reliability solutions for complex engineering systems.
|
|
|
Luis E. Nieto-Barajas ITAM Mexico
|
Title: Markov Processes in Survival Analysis
Abstract: In this talk we present some discrete and continuous Markov processes that have shown to be useful in survival analysis and other biostatistics applications. Both discrete and continuous time processes are used to define Bayesian nonparametric prior distributions. The discrete time processes are constructed via latent variables in a hierarchical fashion, whereas the continuous time processes are based on Lévy increasing additive processes. To avoid discreteness of the implied random distributions, these latter processes are further used as mixing measures of the parameters in a particular kernel, which lead to the so-called Lévy-driven processes. We include univariate and multivariate settings, regression models and cure rate models.
|
|
|
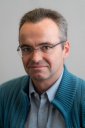
Bruno Tuffin INRIA Rennes France
|
Title: Importance Sampling for the Rare Event Simulation of Reliability Models
Abstract: Monte Carlo simulation methods are often the only tools to get an estimation of performance measures of complex systems. When dealing with the specific class of reliability, we typically require the estimation of probability of order 10^{-9} or even less. It is for example the case of one considers the probability of a failure of nuclear plant, the probability of ruin of an insurance company, the saturation probability in telecommunications… In this case, the crude Monte simulation method, which simply means simulating the system model as many times as possible to obtain the rare event a sufficient number of times is computationally inefficient. Specific methods have been developed in the literature for this rare context, mainly grouped into two classes, importance sampling and importance splitting (also called subset simulation).
During this talk, we are going to review the most efficient application of importance sampling on two types of reliability models: static and dynamic ones. Static models mean that we do not have a stochastic model evolving with time; the system typically has a huge space of states decomposed into two classes, where the system works and where the systems not operational. We often then look at the probability that the systems is down. Dynamic reliability models have components subject to failures and repairs, potentially grouped; we are here interested in the probability of failure of the whole system at a given time, over an interval of time, or the mean time to failure. In each case, we will describe how importance sampling can be applied and discuss the robustness of the estimators with respect to some rarity parameter. We will also discuss the determination of quantile of time to failure, of particular importance for warranty setup for example.
|
|